In the realm of artificial intelligence (AI), there are two predominant methodologies shaping the development landscape: Open-source AI and Closed-source AI respectively. Deep learning and cognitive capabilities approach distinction is a critical factor that defines the evolution of artificial intelligence’s technologies. This document will explore the two disparate concepts of open source and closed source AI and highlight they key differences, the advantages and disadvantages of each and the potential consequences of their usage.
What is Open Source AI? How does IT work
What is known as “open-source AI” or “artificial intelligence” is a term used to describe AI technologies where their source code is published freely to anyone in the public eye. Interpreted thus it implies those developers are able to check, transform and reproduce the code avoiding complicated license agreements alongside they don’t encounter any proprietary barriers. Among the open-source AI frameworks warmly embraced by the AI community, TensorFlow, and Apache MXNet are widely used.
Key Characteristics of Open Source AI:Key Characteristics of Open Source AI:
- Collaborative Development: Open source AI creates a spirit of interaction; contributors with different expertise improve the AI algorithms and models behind it.
- Transparency and Accessibility: Transparency of the open-source AI frameworks allows to get the queries about the algorithms and methodologies that are systems based.
- Community Support: Community of open source developers risks overcoming AI scene through the sharing of convenient solutions, resulting in this way or another the improvements of cutting-edge AI technologies.
- Flexibility and Customization: Providing wide-ranging flexibility, the frameworks fashioned on the open source basis would let developers try things as disparate as computer architecture, algorithms and approaches to optimization.
What is the difference between open source and closed source AI
Licensing and Access:
The central difference between open-source and closed-source AI systems lies in how their licenses are granted and their rights of access. Open-source AI frameworks work under the license of permissive licenses that allow developers the liberty to refer to the source, modify and redistribute it. However, contrary to it, the use of the closed source AI solutions is once again proprietary and involves the use of the binding license agreements that limit the access to the rudimentary code.
Community Collaboration vs. Vendor Control:
AI open source flourishes on community co-creation that refers to the work of many within the common pursuit to improve AI technologies. While, open source AI is driven by the principles of community, collaboration, and empowerment, with the intellectual property of the codes being in the hands of the contributors; closed source AI is tied to the directives and feature sets of products created by vendors.
Customization and Innovation:
Open source AI presents a great chance to build one’s capacity for modified ideas and creation. Developers can be very innovative in algorithms, techniques, and applications they develop. While proprietary AI may have highly the advanced features and functionalities with locking the customization and innovation, it could also create a limit to innovation.
Support and Maintenance:
The power of open-source AI is shared by community-oriented assistance and maintenance. When developers need help, they can reach out for support in online forums, documentation, and collaborative platforms. In contrast, enterprise AI providers originating from closed source AI offer vendor-driven services such as maintenance and support, including speedy issue resolution and optimization of AI deployments on a proactive basis.
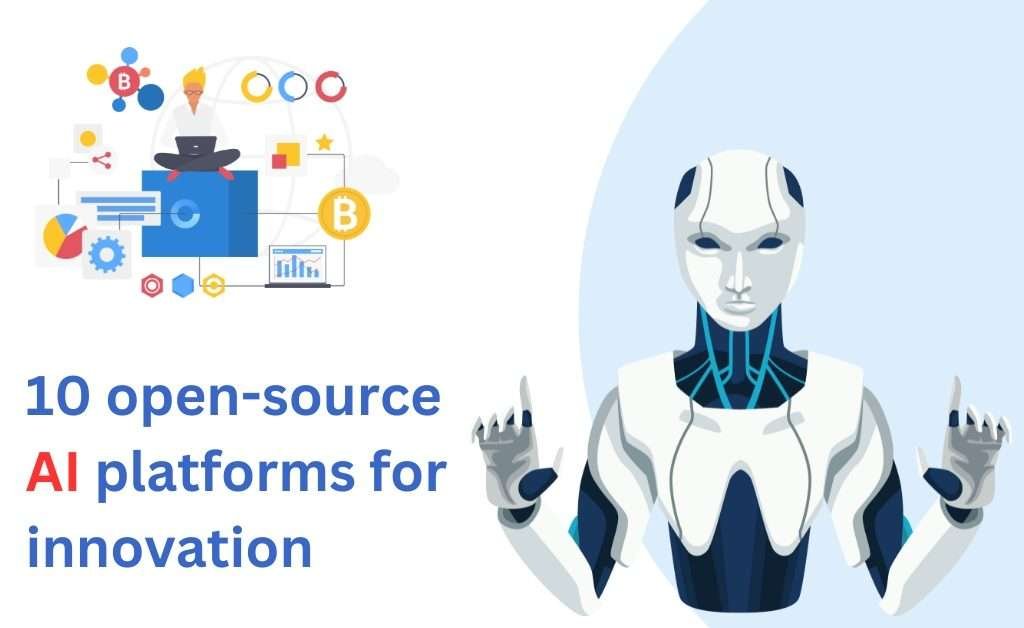
10 open source AI platforms for innovation
AI platforms offer an assortment of AI tools, libraries, and frameworks to be used by developers, AI researchers, or organizations to implement new ideas, cope with different challenges, or produce innovative products. Open source AI platform brings together machine learning algorithms, natural language processing models, and other tools to generate applications that can be built, trained, and deployed to diffuse across the broad areas of industries, where they are extensively used in various application scenarios.
1. TensorFlow: open source AI platforms for innovation
TensorFlow, being the backbone of artificial intelligence ecosystem built by Google Brain, is one of the most convenient open source platforms, having an abundant set functions for the development of models, their training and final deployment.
Features:
- Scalable: TensorFlow, a compute infrastructure with decentralized framework, can connect single device to larger distributed systems with no hindrance.
- Flexibility: With the modular structure of TensorFlow being transparent, experimenting and customization are achieved in an easy way, making this framework the good choice for both research and production environments.
Pros:
- Extensive Community Support: TensorFlow not only possesses a very active community of developers and scientists, but it also offers various useful training tools in the shape of tutorials, models, and project protocols.
- Integration: TensorFlow can be effortlessly combined within your favorite programming languages such as Python, Java or even TensorFlow Serving.
Cons:
- Steep Learning Curve: The intricate nature of TensorFlow architecture can be rather challenging to grasp even the first-time learners.
2. H2O: open source AI platforms for innovation
Among many other things, H2O, an AI platform developed under an open source license, is elegant because of a rich list of features and the make-it-easy interface, which serves those looking for a perfect tool for all those looking for the ideal AI tool out there.
Features:
- Distributed Computing: The H2O platform incorporates parallel processing which enables it to take care of even more complicated computations of huge scale on arrays of machines.
- AutoML: H2O’s AutoML, a smart algorithm, makes the machine learning pipeline, like data preprocessing, model selection, and tuning, extra automatic, reducing the model development process complication.
Pros:
- Ease of Use: Being user-friendly, H2O is of course supported by well-designed interface and detailed documentation which anyone with any skills level could read and learn from.
3. DALL-E: open source AI platforms for innovation
DALL-E, the breakthrough open source AI platform pioneered by OpenAI, discloses the future of our AI based creativity. It’s amazing to find that the system can create visual artworks based on a natural generated text.
Features:
- Image Generation: With DALL-E, text-based information is generated with the most recent deep learning tools. As a result, users can create life-like visual images to support their idea.
- Customization: DALL-E also offers the possibility for users to adjust different parameters for the output, such as style, composition, and level of detail so it is in alignment with the user preferences and needs.
Pros:
- Creative Potential: DALL-E enables artists to pursue the creation of inspired works and open new territories of expression allowing users to produce sophisticated and feasible pictures autonomously.
- Accessibility: As an open-source project, DALL-E is easily accessible by the public, so it becomes an instrument for bringing together and further developing the AI community.
Cons:
- Resource Intensive: Producing images meeting high standards of DAL-E probe can be expensive in terms of computing power and time.
4. Rasa: open source AI platforms for innovation
Rasa, Open source AI platforms for the spread of ideas and innovation become a tool which helps scientists and other thinkers around the planet. Rasa, a conversational AI platform open source, enables developers to make highly intelligent chatbots and personal assistants with natural language processing functionality.
Features:
- Natural Language Processing: Rasa NLP enhancement allows the chatbots to comprehend and respond to users’ inquiries in natural language, so that the user engagement in the conversation is provided and enhanced.
- Machine Learning: Rasa uses machine learning approaches to generate a feedback loop on chat bot performance based on the interactions of the user the, system can fine-tune itself over time this way it become increasingly a personalized and contextually relevant chatbot.
Pros:
- Customization: Rasa allows the users to have amazing customization options. The developers can go into the depths of the behaviors, utterance, and workflow to reflect the business needs better.
- Community Support: Rasa has a very strong community of developers and contributors that helps to support its users by providing them with resources, tutorials, and support to expedite the process of a building a chatbot and help with deployment.
Cons:
- Learning Curve: Learning Rasa well with its advancement features and customization abilities could require one more time and skill set to finish it.
5. ChatGPT: open source AI platforms for innovation
ChatGPT is OpenAI’s open-source talk AI model that is based on chatbot scenes. It provides the developers with the opportunity of creating incredible chatbots along with conversational interfaces due to its ability to understand natural language processing.
Features:
- Natural Language Processing: Chat GPT employs the mark-up of the latest transformer architecture for understanding the text and generating the human like response thereby creating engaging and contextually deepening conversations.
- Customizability: The developers of ChatGPT can adjust the system’s behavior, mood and the response of the agents, so that they are aligned with the specific needs of the business and the preferences of the user to ensure a highly personalized and tailored conversational experience.
Pros:
- Versatility: ChatGPT is quite flexible and can be used for a variety of different conversational applications such as providing customer support, virtual assistants and interactive storytelling.
- Accessibility: ChatGPT is being developed as an open-source project with an open mission to convene developers, which in turn will promote further collaboration and innovation among the AI community.
Cons:
- Training Data Requirements: Achieving chatbot performance that could be true to the human level could be reached by investing in a huge amount of training data and computing resources.
6. OpenCV: open source AI platforms for innovation
OpenCV, the free and open source computer vision library, is the heart of the artificial intelligence field and assists developers in their creation of advanced computer vision applications with great ease.
Features:
- Cross-Platform Compatibility: OpenCV is compatible with multiple programming languages lies in the integration and development communities whose members can choose between C++, Python, and Java.
- Real-Time Processing: It is just a great benefit of the optimized algorithms taking data structures into account within OpenCV that real-time image and video processes are made possible. Consequently, applications are able to be emerged in more fields such as robotics, surveillance, and augmented reality.
Pros:
- Accessibility: OpenCV operates under the BSD license syntax, thus giving the user the ability to access, modify and redestribute all of the OpenCV library features freely.
- Extensive Documentation: OpenCV comes with an extensive and detailed documentation and a pool of tutorials and examples that aid the developers even at the basic level and advanced level.
Cons:
- Steep Learning Curve: It is of much importance to ensure the use of depth perception tools like OpenCV in order to prevent accidents or injuries on the road.
7. Keras: open source AI platforms for innovation
Keras, the open source deep learning framework which offers user laptop for developing and training neural models user friendly, completely revolutionizes artificial intelligence development by providing building and neural networks with ease.
Features:
- Modular Architecture: One distinctive feature of Keras is its modular architecture which results in easy integration with other deep learning frameworks including TensorFlow and Theano for the designs to be used for flexibility and interoperability purposes.
- Extensive Library: Keras includes a large diversity of ready-made building blocks including layers, models, and utilities used for typical deep learning tasks, allowing developers to easily work with them.
Pros:
- Ease of Use: Features such as Keras’s easy to use and intuitive API, which makes prototype, exploration, and model deployment faster, is what makes it such an appealing deep learning framework.
- Flexibility: Keras inbuilt modular architecture is greatly flexible for customization and some extensions too; therefore developers can give model parameters for specific requirements and suitable use-cases.
Cons:
- Limited Low-Level Control: The use of API (Application Protocol Interface) in Keras (a library of neural networks for Python) facilitates the creation of complex models but allows no low-level control over architecture and the strategies of optimization.
8. Apache MXNet: open source AI platforms for innovation
Keras with Apache MXNet back-end, the Keras framework that boasts about the simplicity and ease of use with the scalability and performance of Apache MXNet, is a powerful solution for developing and implementing deep learning models.
Features:
- User-Friendly Interface: Keras comes with easy to use, high-level API that help runs neural networks accordingly as they facilitate simple task of building and training neural networks, on the other hand, Apache MXNet provides scalable and efficient operation for handling massive data and complex models.
- Extensive Functionality: The linking of Keras and Apache MXNet allows to use the libraries that include any of the most commonly used models, layers, and tools for the purpose of deep learning tasks. This aids the speedy product designing and trials.
Pros:
- Ease of Use: Keras’s user-friendly API and the scalability of Apache MXNet as a back-end environment helps in the website development for new as well advance level web developers making the experience versatile.
- Performance: The MXNet computing performance from, Apache MXnet, coupled with the its distributed training can make deep learning tasks highly performant and scalable.
Cons:
- Integration Complexity: In regards to configuring Keras with the Apache MXNet backend, there is a high level on effort that is comparative if one is a stand alone framework users.
9. Theano: open source AI platforms for innovation
Theano, an open source deep learning library found to be highly effective in computationally intensive deep learning tasks and is quite flexible, has been an important instrument of scientific advancement in the field of AI.
Features:
- Symbolic Expression: Theano provides the functionality of symbolic programming which enables automatic differentiation and optimization methods for gradients computations that are input data-driven generally.
- GPU Acceleration: Theano can take advantage of GPU acceleration, querying GPUs in order to speed up the computation and training of deep learning models.
Pros:
- Efficiency: Theano’s symbolic expression evaluation and GPU acceleration performance allows a higher degree of model training and performance in practice.
- Flexibility: With its, Theano is an extensible package that is programmer friendly and readily suitable to use-cases-specific algorithms and optimizations.
Cons:
- Learning Curve: The symbolic expression syntax of theano may be long for learners, that can require the knowledge of basic technical issues and programming principles.
10. IBM: open source AI platforms for innovation
IBM, a leader in technology and innovation, offers a suite of open source AI platforms that empower developers and organizations to drive advancements in artificial intelligence.
Features:
- Watson Studio: IBM Watson Studio provides a collaborative environment for data scientists and developers to build, train, and deploy machine learning models.
- Watson Machine Learning: IBM Watson Machine Learning offers scalable machine learning capabilities, enabling users to deploy models in the cloud or on-premises.
Pros:
- Enterprise-Grade Solutions: IBM’s AI platforms offer enterprise-grade features and support, making them suitable for large-scale deployments and mission-critical applications.
- Integration: IBM’s AI platforms seamlessly integrate with other IBM Cloud services and solutions, as well as third-party tools and frameworks.
Cons:
- Cost: IBM’s enterprise-grade solutions may come with a higher price tag compared to some open source alternatives.
Conclusion
Open source AI promotes joint efforts and transparency, making the developers benefit because of their freedom and your community capacity to help you. It’s as if a gathering of receptive minds where you can both put forth your views and draw from the ideas of others also on the other hand, closed source AI offers the advantage of unique features and superior support, but sacrifice flexibility and visibility of the solution. It’s akin to a well-fitted tailored suit, where the aesthetic fits are razor sharp, but you may find it harder to adjust to your style needs. Finally, while you ponder between open source and closed source AI, you must consider the issues that matter to you most and as well anticipate the exact needs of your project.